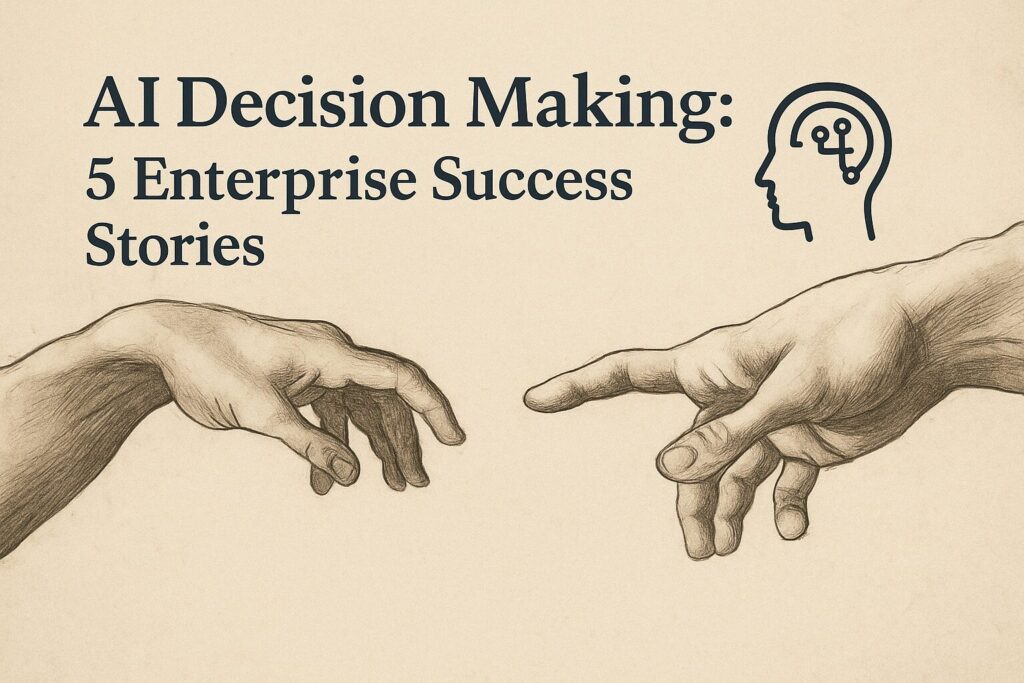
AI and Decision Making
Defining AI Decision Making
AI decision making leverages machine learning, natural language processing, and predictive analytics to process vast datasets, identify patterns, and recommend actions with minimal human intervention. Unlike traditional methods reliant on experience or limited data, AI offers real-time insights, enabling enterprises to navigate complex challenges in areas like customer service, supply chain management, and strategic planning.
The Rise of AI in Enterprises
AI adoption has grown significantly, with IBM research indicating that 42% of enterprises with over 1,000 employees used AI in 2024, up from 37% in 2019 and 10% in 2015. This trajectory suggests continued growth in 2025, driven by AI’s ability to deliver competitive advantages. McKinsey projects AI could add $13 trillion to global economic output by 2030, boosting GDP by 1.2% annually.
From Gut Feel to AI Decision Making
The Shift to Data-Driven Decisions
Historically, business leaders relied on intuition or “gut feel” for decisions, especially under time constraints or data scarcity. However, this approach often led to inconsistencies. AI transforms this paradigm by providing data-driven insights, reducing decision stress, and enhancing accuracy. A Harvard Business Review study notes that 85% of leaders experience decision stress, with 75% facing a tenfold increase in daily decisions over three years. AI alleviates this by offering predictive models and real-time analytics.
Benefits of AI-Driven Decisions
AI enables faster, more accurate decisions by:
- Analyzing Large Datasets: Processing terabytes of data to uncover trends.
- Predicting Outcomes: Analyzing and foreseeing market shifts or customer behavior.
- Reducing Bias: Minimizing human error, though AI models require careful design to avoid inherent biases.
- Enhancing Speed: Augmenting decisions helping leaders in strategic tasks.
This shift is critical in high-pressure environments, where AI acts as a decision-making partner, as highlighted by PwC’s 2025 AI Predictions, which emphasize AI’s role in achieving strategic objectives and strong ROI.
Case Studies
Case Study 1: Nationwide
Overview: Nationwide, a leading UK insurance and financial services provider with 17 million customers, sought to improve customer query handling efficiency.
Challenge: Generating response letters was time-consuming, averaging 45 minutes per query, straining resources.
AI Solution: Nationwide implemented GPT-4 within Azure OpenAI Service to automate letter generation, supported by a responsible AI framework ensuring human review.
Results: Response times dropped to 10-15 minutes, a 66% efficiency gain, allowing staff to handle more queries and focus on complex issues.
Impact on Decision Making: Faster responses enabled customer service teams to make quicker, more informed decisions, enhancing customer satisfaction and operational efficiency.
Case Study 2: Pegasus Airlines
Overview: Pegasus Airlines, a Turkish low-cost carrier, aimed to enhance customer support and internal operations.
Challenge: High query volumes and internal process inefficiencies required scalable solutions.
AI Solution: Using Azure OpenAI and Azure AI Services, Pegasus deployed generative AI chatbots like FlyBot for customers and Hero for employees, supporting nearly 100 use cases.
Results: Customer satisfaction doubled, and employee satisfaction rose by 20%, with 3,000 employees using Hero monthly.
Impact on Decision Making: AI chatbots provided rapid, accurate responses, aiding customers in booking decisions and employees in operational choices, streamlining workflows.
Case Study 3: UniSuper
Overview: UniSuper, an Australian superannuation fund, focused on improving operational efficiency to better serve members.
Challenge: Summarizing client interactions was time-intensive, diverting resources from strategic tasks.
AI Solution: UniSuper adopted Microsoft 365 Copilot, scaling from 300 to 1,200 licenses in 2024, to automate interaction summaries.
Results: Saved 30 minutes per interaction, projecting 1,700 hours annually, and increased advised members by 7%.
Impact on Decision Making: By reducing administrative burdens, AI enabled staff to prioritize strategic decisions, enhancing member outcomes.
Case Study 4: StarKist Foods
Overview: StarKist Foods, a global food manufacturer, needed to streamline production and demand planning.
Challenge: Planning cycles took 16 hours, hindering agility.
AI Solution: StarKist implemented the Board Intelligent Planning Platform on Azure, integrating Azure Machine Learning for forecasting.
Results: Planning cycles reduced to under one hour, a 94% improvement, with consistent forecast accuracy.
Impact on Decision Making: Faster planning allowed real-time, data-driven decisions, improving resource allocation and market responsiveness.
Case Study 5: TMBThanachart (ttb)
Overview: TMBThanachart Bank (ttb), a major Thai bank, aimed to enhance customer service efficiency.
Challenge: High contact center usage strained resources and response times.
AI Solution: ttb developed the ‘Yindee’ virtual assistant using Azure OpenAI Service, handling queries in Thai and English.
Results: Reduced contact center usage by 10% and achieved an 80% satisfaction score.
Impact on Decision Making: AI-driven query resolution enabled faster customer service decisions, improving client experiences and operational efficiency.
Risks & Governance in AI Decision Making
Potential Risks
AI in decision making introduces risks that require proactive management:
- Bias and Fairness: AI models may perpetuate biases if trained on skewed data.
- Transparency: Lack of explainability can erode trust in AI outputs.
- Data Privacy: Handling sensitive data raises compliance concerns.
- Over-Reliance: Excessive dependence on AI may diminish human expertise.
Governance Frameworks
To address these risks, enterprises must adopt robust governance:
- Ethical Guidelines: Establish principles for fair and transparent AI use.
- Regular Audits: Monitor AI systems for bias and performance issues.
- Human Oversight: Ensure human review of critical AI decisions, as practiced by Nationwide.
- Regulatory Compliance: Align with evolving state and federal regulations, as noted by PwC.
PwC’s 2025 predictions emphasize that successful AI governance balances risk mitigation with strategic objectives, ensuring trust and ROI.
90-Day Adoption Playbook
To integrate AI into decision making within 90 days, enterprises can follow this structured plan:
- Days 1-15: Identify Key Focus Areas
- Pinpoint decision-making areas like strategy and marketing organizations that AI can enhance.
- Engage stakeholders to align AI goals with business strategy.
- Days 16-30: Assess Data Readiness
- Evaluate data quality, availability, and accessibility.
- Cleanse data to ensure accuracy for AI models.
- Days 31-45: Select AI Tools
- Choose platforms like NexStrat.AI based on scalability and integration needs.
- Consider vendor support and ease of deployment.
- Days 46-60: Pilot AI Solutions
- Implement AI in a controlled environment, testing on a single domain.
- Collect feedback to refine strategic approach and operating model.
- Days 61-75: Train Staff
- Conduct training on AI tool usage and interpretation of insights.
- Foster a culture of AI literacy and collaboration.
- Days 76-90: Monitor and Evaluate
- Track AI performance metrics like efficiency gains and decision accuracy.
- Adjust strategies to optimize outcomes and scale adoption.
Table 2: 90-Day AI Adoption Playbook
Phase | Days | Action | Key Activities |
---|---|---|---|
1 | 1-15 | Identify Domains | Stakeholder alignment, domain selection |
2 | 16-30 | Assess Data | Data quality evaluation, cleansing |
3 | 31-45 | Select Tools | Platform evaluation, vendor selection |
4 | 46-60 | Pilot Solutions | Controlled testing, feedback collection |
5 | 61-75 | Train Staff | Training programs, cultural integration |
6 | 76-90 | Monitor & Evaluate | Performance tracking, strategy optimization |
Next Steps: Deploy with NexStrat AI
For enterprises seeking to harness AI for strategic decision making, NexStrat.AI offers a robust platform tailored to 2025’s demands. Its AI-driven tools accelerate insight generation, fast and scalable framework based strategy generation, and facilitate cross-functional collaboration. NexStrat.AI’s five-step framework—Assess, Analyze, Collaborate, Mitigate, Execute—guides businesses through:
- Assess: Defining challenges and building data-driven hypotheses.
- Analyze: Testing strategic options and quantifying outcomes.
- Collaborate: Enabling fast and at-scale team alignment.
- Mitigate: Identifying and managing risks proactively.
- Execute: Creating actionable roadmaps with clear milestones.
By leveraging NexStrat.AI, enterprises can emulate the success of the showcased case studies, achieving measurable ROI and competitive advantage. Book a demo to explore how NexStrat.AI can transform your decision-making processes.
About NexStrat AI:
NexStrat AI is at the forefront of AI and business strategy innovation. As the ultimate strategy and transformation AI co-pilot and platform, we help leaders and strategists craft winning strategies and make effective decisions with speed and confidence.
Contact Us:
Have questions or want to learn more? Contact us at info@nexstrat.ai
Follow Us:
Join our community of forward-thinking business leaders on LinkedIn.